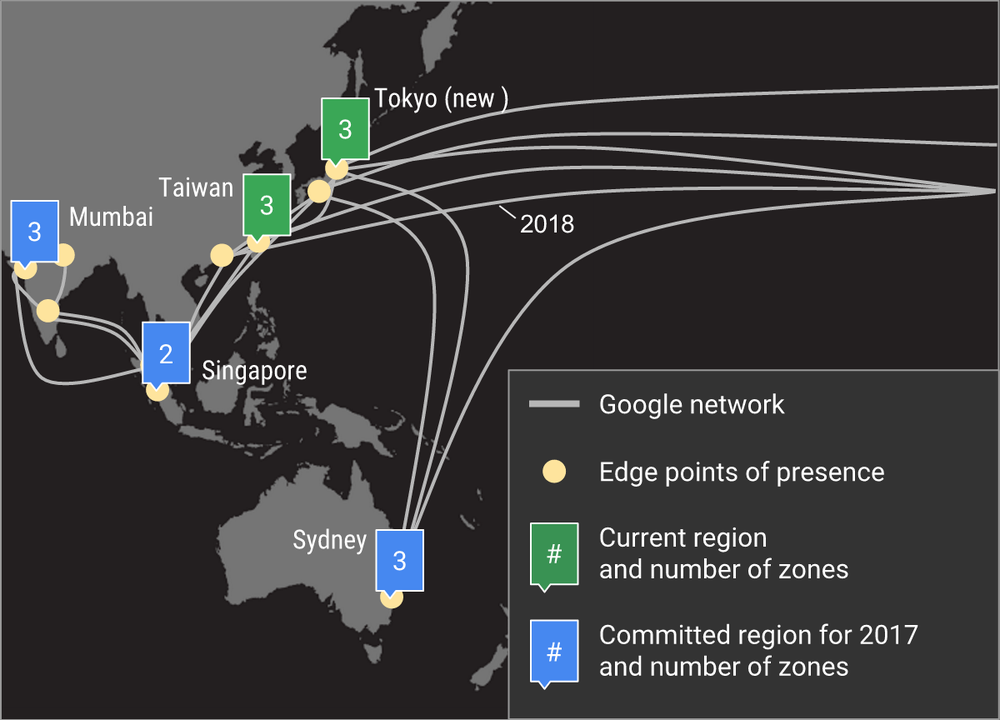
Google invited 13000 programmers and to its largest Asia pacific cloud event.
Google Cloud Next Tokyo. During this event, we celebrated the many ways that Japanese companies such as Kewpie, Sony (and even cucumber farmers) have transformed and scaled their businesses using Google Cloud.
Since the launch of the Google Cloud Tokyo region last November, roughly 40 percent of Google Compute Engine core hour usage in Tokyo is from customers new to Google Cloud Platform (GCP). The number of new customers using Compute Engine has increased by an average of 21 percent monthly over the last three months, and the total number of paid customers in Japan has increased by 70 percent over the last year.
By supplying compliance statements and documents for FISC — an important Japanese compliance standard — for both GCP and G Suite, we’re making it easier to do business with Google Cloud in Japan.
Here are a few of the exciting announcements that came out of Next Tokyo:
Retailers embracing enterprise innovation
One of the biggest retailers in Japan, FamilyMart, will work with Google’s Professional Services Organization to transform the way it works, reform its store operations, and build a retail model for the next generation. FamilyMart is using G Suite to facilitate a collaborative culture and transform its business to embrace an ever-changing landscape. Furthermore, it plans to use big data analysis and machine learning to develop new ways of managing store operations. The project, — dubbed “Famima 10x” — kicks off by introducing G Suite to facilitate a more flexible work style and encourage a more collaborative, innovative culture.
Modernizing food production with cloud computing, data analytics and machine learning
Kewpie, a major food manufacturer in Japan famous for their mayonnaise, takes high standards of food production seriously. For its baby food, it used to depend on human eyes to evaluate 4 – 5 tons of food materials daily, per factory, to root out bad potato cubes — a labor-intensive task that required intense focus on the production line. But over the course of six months, Kewpie has tested CloudMachine Learning Engine and TensorFlow to help identify the bad cubes. The results of the tests were so successful that Kewpie adopted the technology.
Empowering employees to conduct effective data analysis
Sony Network Communications Inc. is a division of Sony Group that develops and operates cloud services and applications for Sony group companies. It converted from Hive/Hadoop to BigQuery and established a data analysis platform based on BigQuery, called Private Data Management Platform. This not only reduces data preparation and maintenance costs, but also allows a wide range of employees — from data scientists to those who are only familiar with SQL — to conduct effective data analysis, which in turn made its data-driven business more productive than before.
Collaborating with partners
During Next Tokyo, we announced five new Japanese partners that will help Google Cloud better serve customers.
- NTT Communications Corporation is a respected Japanese cloud solution provider and new Google Cloud partner that helps enterprises worldwide optimize their information and communications technology environments. GCP will connect with NTT Communications’ Enterprise Cloud, and NTT Communications plans to develop new services utilizing Google Cloud’s big data analysis and machine intelligence solutions. NTT Communications will use both G Suite and GCP to run its own business and will use its experiences to help both Japanese and international enterprises.
- KDDI is already a key partner for G Suite and Chrome devices and will offer GCP to the Japanese market this summer, in addition to an expanded networking partnership.
- Softbank has been a G Suite partner since 2011 and will expand the collaboration with Google Cloud to include solutions utilizing GCP in its offerings. As part of the collaboration, Softbank plans to link GCP with its own “White Cloud” service in addition to promoting next-generation workplaces with G Suite.
- SORACOM, which uses cellular and LoRaWAN networks to provide connectivity for IoT devices, announced two new integrations with GCP. SORACOM Beam, its data transfer support service, now supports Google Cloud IoT Core, and SORACOM Funnel, its cloud resource adapter service, enables constrained devices to send messages to Google Cloud Pub/Sub. This means that a small, battery-powered sensor can keep sending data to GCP by LoRaWAN for months, for example.
Create Cloud Spanner instances in Tokyo
Cloud Spanner is the world’s first horizontally-scalable and strongly-consistent relational database service. It became generally available in May, delivering long-term value for our customers with mission-critical applications in the cloud, including customer authentication systems, business-transaction and inventory-management systems, and high-volume media systems that require low latency and high throughput. Starting today, customers can store data and create Spanner instances directly in our Tokyo region.
Jamboard coming to Japan in 2018
At Next Tokyo, businesses discussed how they can use technology to improve productivity, and make it easier for employees to work together. Jamboard, a digital whiteboard designed specifically for the cloud, allows employees to sketch their ideas whiteboard-style on a brilliant 4k display, and drop images, add notes and pull things directly from the web while they collaborate with team members from anywhere. This week, we announced that Jamboard will be generally available in Japan in 2018.
Why Japanese companies are choosing Google Cloud
For Kewpie, Sony and FamilyMart, Google’s track record building secure infrastructure all over the world was an important consideration for their move to Google Cloud. From energy-efficient data centers to custom servers to custom networking gear to a software-defined global backbone to specialized ASICs for machine learning, Google has been living cloud at scale for more than 15 years—and we bring all of it to bear in Google Cloud.
We hope to see many of you as we go on the road to meet with customers and partners, and encourage you to learn more about upcoming Google Cloud events.
Recent Comments